How to Use an AI Server for Your Business
Mar 13th 2025
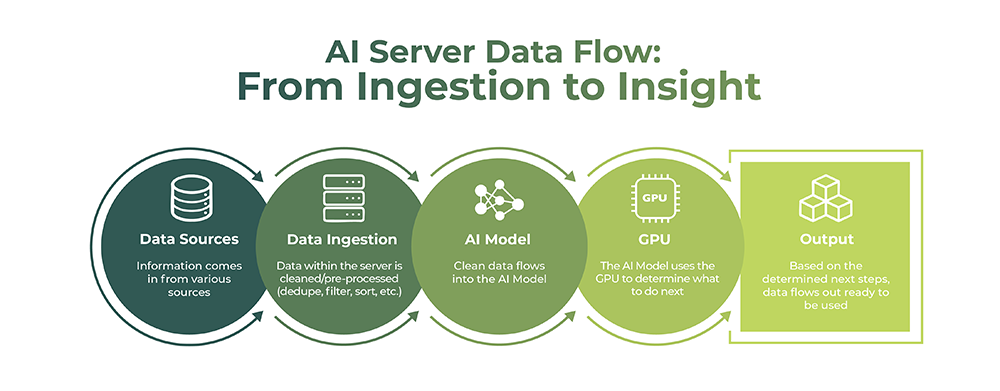
What is AI and Machine Learning in Server Environments?
AI and Machine Learning involve sophisticated algorithms that can learn from and make decisions on data. Here's a small list of examples on how they integrate with server technology:
-
Process Large Datasets: AI servers are designed to handle massive volumes of data for purposes like analysis, model training, or real-time decision-making. They are equipped with high-speed storage and I/O capabilities to manage data throughput efficiently, ensuring that complex tasks can be processed quickly and accurately.
-
Enable Advanced Computing: These servers perform calculations that traditional computing might struggle with in terms of time or resource demands. With powerful CPUs and specialized GPUs, AI servers accelerate computations necessary for deep learning, neural networks, and other AI methodologies, significantly reducing processing times for tasks like image recognition, natural language processing, or predictive analytics.
-
Support Automation: From automating repetitive tasks to predicting future maintenance requirements, AI servers empower businesses to automate operations at a large scale. They can run algorithms that continuously learn from operational data to optimize processes, enhance security through anomaly detection, or automate customer interactions through chatbots and virtual assistants, thereby increasing efficiency and reducing human error.
Who Uses AI Servers?
-
Enterprises:
-
For enhancing customer relationship management (CRM) with predictive analytics to tailor marketing strategies or anticipate customer needs.
-
In supply chain management to optimize logistics, predict demand, and manage inventory more effectively.
-
Research Institutions:
-
Universities and research labs use AI servers for academic research in fields like astrophysics for modeling galaxy formations or in biology for protein folding studies.
-
For climate modeling to predict weather patterns or simulate environmental impacts over time.
-
Healthcare:
-
Hospitals and clinics leverage AI servers for diagnostic support, where algorithms help in analyzing medical images for early detection of diseases like cancer or heart conditions.
-
In developing personalized medicine by analyzing patient genetic data to tailor treatments.
-
Finance:
-
Banks and financial institutions employ AI servers for real-time fraud detection systems that learn from transaction patterns.
-
For high-frequency trading where algorithms make rapid buy/sell decisions based on market data analysis.
-
Manufacturing:
-
For predictive maintenance, where AI servers analyze sensor data from machinery to predict failures before they occur, thus reducing downtime.
-
In quality control, where AI visually inspects products on the assembly line for defects.
-
Retail:
-
To analyze consumer behavior for dynamic pricing strategies or to optimize store layouts based on customer flow analysis.
-
For inventory management, predicting stock levels with demand forecasting models.
-
Automotive:
-
In the development of autonomous vehicles, where AI servers process vast amounts of data from test drives to improve self-driving algorithms.
-
For smart manufacturing processes, enhancing the precision and efficiency of vehicle production.
-
Media and Entertainment:
-
For content recommendation engines that personalize user experiences on streaming platforms.
-
In video game development, where AI can generate dynamic environments or NPC behaviors.
-
Government and Public Sector:
-
For urban planning, using AI to model traffic flow and optimize public transport systems.
-
In defense, AI servers can be used for intelligence analysis or in cybersecurity operations to detect and respond to threats.
Scalability Considerations for AI and ML Environments
As your business grows or your AI projects evolve, scalability becomes a critical factor in maintaining performance and efficiency. Here’s what to consider:
Hardware Growth: Choose servers and workstations that allow for easy upgrades, such as additional GPUs, RAM, or storage. Systems like the Dell PowerEdge R650 or Lenovo ThinkStation P920 are designed with expansion in mind, enabling you to scale compute power as your needs increase.
Distributed Computing: For larger AI workloads, scalability often means moving beyond a single server to a cluster. High-speed networking (e.g., 25GbE or 100GbE) and software frameworks like Apache Spark or Kubernetes can distribute tasks across multiple machines, enhancing throughput and resilience.
Cloud Hybrid Options: Integrating on-premises hardware with cloud resources offers flexibility. For instance, during peak demand, you can offload processing to cloud platforms like AWS or Azure, scaling capacity without immediate hardware investments.
Workload Optimization: Plan for scalability by profiling your AI workloads. Low-tier options like the HP Z4 G4 might suffice initially, but transitioning to medium or high-tier solutions (e.g., HPE ProLiant DL360 Gen10 Plus or Dell Precision 7920) ensures you’re ready for more complex models or larger datasets.
Scalability isn’t just about adding hardware; it’s about building a system that adapts to your business’s AI ambitions, balancing cost, performance, and future-proofing.
Important Server Specifications for AI and Machine Learning:
-
CPU (Central Processing Unit):
-
Core Count: High core counts are essential for parallel processing, allowing for simultaneous handling of multiple AI tasks or threads. Look for servers with newer generations of Intel Xeon or AMD EPYC processors which offer high core counts.
-
Clock Speed: While core count is crucial, clock speed also matters for tasks that aren't fully parallelizable. Higher clock speeds can accelerate single-threaded computations within AI workloads.
-
GPU (Graphics Processing Unit):
-
CUDA Cores (for NVIDIA) or Compute Units (for AMD): These are the workhorses for deep learning, providing massive parallel computation capabilities. More cores or units mean better performance for tasks like training neural networks or running inference.
-
Memory: GPU memory (VRAM) is critical for handling large models and datasets. At least 16GB per GPU is recommended, with 32GB or more preferred for cutting-edge AI work.
-
Tensor Cores: Specialized hardware like NVIDIA's Tensor Cores can accelerate matrix multiplication, a common operation in deep learning, offering significant speed-ups.
-
Memory (RAM):
-
Capacity: AI models, especially during training, can require vast amounts of RAM. Aim for at least 256GB, but for more complex models or larger datasets, 512GB or more might be necessary.
-
Speed: Higher memory bandwidth (e.g., DDR4 at 3200MHz or higher) ensures that data can be fed to processors quickly, reducing bottlenecks.
-
Storage:
-
Type: SSDs (Solid State Drives) are a must for AI due to their speed. NVMe SSDs offer even faster data access, crucial for quick model loading and data retrieval.
-
Capacity and Configuration: Balance between speed (throughput) and capacity. RAID configurations can offer both performance boosts and data redundancy. For vast datasets, consider scale-out storage solutions.
-
I/O Performance: High IOPS (Input/Output Operations Per Second) are vital for dealing with the frequent read/write operations during training sessions.
-
Networking:
-
Bandwidth: High-speed networking (10GbE or higher) is essential for data transfer between servers in a cluster or to external storage or cloud services, particularly for distributed AI training.
-
Latency: Low latency is key for real-time AI applications or when coordinating between multiple nodes in a high-performance computing environment.
Recommended AI and Machine Learning Servers
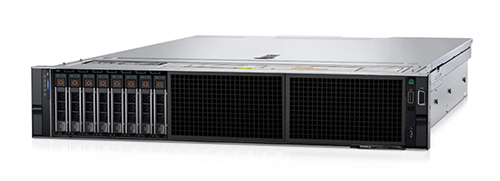
Dell AI Servers - Dell PowerEdge R750
-
Specs: Supports 3rd Gen Intel® Xeon® Scalable processors, up to 3TB of DDR4 memory, and multiple GPU options for acceleration.
-
Use Case: Ideal for enterprises needing robust performance for AI model training and deployment with significant data processing demands.
Dell AI Servers - Dell PowerEdge R650
-
Specs: Supports 3rd Gen Intel® Xeon® Scalable processors, up to 3TB of DDR4 memory, and multiple GPU options for acceleration.
-
Use Case: Ideal for enterprises needing robust performance for AI model training and deployment with significant data processing demands.
HPE AI Servers - HPE ProLiant DL360 Gen10 Plus
-
Specs: Comes with 3rd Gen Intel® Xeon® Scalable processors, up to 1TB of DDR4 memory, and supports up to 3 GPUs for AI workloads.
-
Use Case: Suitable for medium-sized businesses or departments within larger organizations looking to leverage AI with a balance between cost and performance.
Dell AI Servers - Dell PowerEdge R740
-
Specs: Equipped with Intel® Xeon® Scalable processors, up to 3TB of memory, and versatile storage options, though with less GPU support compared to higher tiers.
-
Use Case: Perfect for small businesses or startups beginning to explore AI applications, offering a cost-effective entry point into AI server technology.
Recommended AI and Machine Learning Workstations
Lenovo AI Workstations - Lenovo ThinkStation P920
-
Specs: Dual Intel Xeon processors, up to 3TB memory, and support for multiple high-end graphics cards for intensive AI and ML tasks.
-
Use Case: Best for professionals in data science, engineering, or research requiring top-tier performance for model development and simulation.
HP Dell Workstations- Dell Precision 7920
-
Specs: Similar to the P920 but might offer slightly different GPU options and less memory capacity, still highly capable for AI workloads.
-
Use Case: A go-to for companies needing powerful workstations for machine learning but with a budget constraint compared to the highest tier.
HP AI Workstations - HP Z4 G4
-
Specs: A single-socket workstation with up to 128GB of RAM, designed for entry-level AI tasks with support for one professional GPU.
-
Use Case: Ideal for small teams or individual developers working on AI projects, or educational settings where budget and space are limited.
In conclusion, selecting the right hardware for AI and Machine Learning can significantly impact your project's success. Whether you're a large corporation or a small startup, there's a tier of server and workstation tailored to your needs, ensuring you can harness the power of AI to drive your business forward. Remember, the choice between these options should align with your current data demands, scalability plans, and, importantly, your budget. Many businesses struggle to find the right balance between performance and cost when investing in AI infrastructure. At PCSP, we provide a solution: high-quality, refurbished servers and workstations backed by expert technical support. We help you overcome budget constraints and scale your AI capabilities with confidence. Discover how PCSP can empower your AI journey – visit our website or contact us for a personalized consultation.
Disclosure: PCSP is the author and publisher of this blog post.